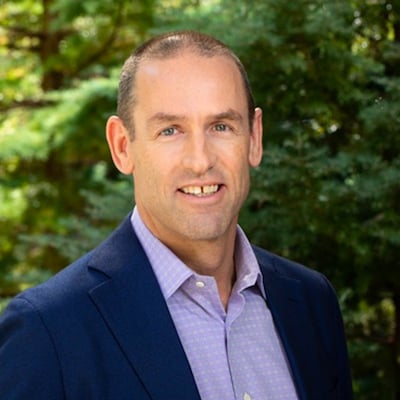
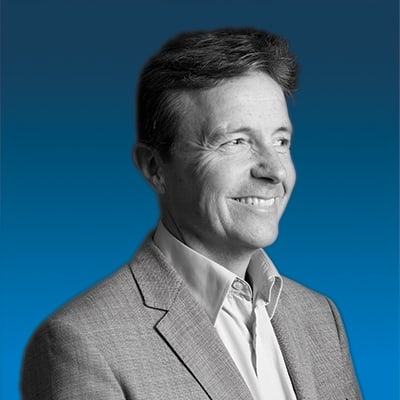
Alex Boyd & Phil Shepherd
PSC and partner Harmonic Analytics are headed to GridFWD Seattle on April 19-20 to take part in a two-day energy transition deep dive. In this blog post, PSC CEO Alex Boyd and Harmonic Analytics CEO Phil Shepherd share a sneak peek into their joint conference presentation. Get a preview as they contemplate how utilities can maximize the value of their data by distinguishing the relationship between data quality and analytics.
Data volume growth is off the charts
Electricity utilities now manage more data than ever before, generated from complex network control systems and the deployment of smart meters and asset condition-monitoring devices.
As the incoming telemetry from these devices increases, the management of these data becomes increasingly important so that trends and indicators can be easily distinguished from data at scale. The volume and diversity of the data can make it difficult for non-technical business users and managers to assess the usefulness of data for analytics purposes.
Analytics services are available which can help to automate the assessment of big data and provide advice and services to remediate, manage, and extract value from the data using machine learning and other powerful analytical techniques.
Garbage in. Garbage out.
The benefits that analytics initiatives can deliver are directly correlated to the quality of the underlying data. Manual or automated data cleaning improves the predictive power of analytics and delivers outcomes that support insights with less uncertainty leading to a clearer understanding of business risks and the financial impact of different decisions.
For example, asset condition survey data are often not regularly audited leading to poor inputs to asset lifecycle analysis and planning. An automated data quality tool can help to identify limitations in these data, such as missingness, outliers and likely erroneous data.
A confidence grade can be assigned at the record level using domain knowledge and historical data, enabling data customers to clearly define assumptions and build trusted analytics products.
Data connectivity creates opportunities
While the benefits of digitally collecting data are clear, the value extracted using predictive analytics can be diminished through poor data quality practices. For example, an investment in an individual system can be significant, but failing to integrate this into existing business platforms can be costly.
Another aspect of data quality is the capacity for data from different business systems to be combined to be used in the analysis. Integrated systems which share common dimensions across business datasets lead to a richer set of data to be mined for analytics.
By integrating an ADMS system to GIS, the operations team is provided the tools to analyze and simulate spatial risk scenarios to optimize switching procedures. Adding integration to an asset management system can provide engineering teams with near real-time failure statistics to drive deterioration and replacement modeling.
Data as an asset, not a liability
Data assets that are utilized, curated and maintained return value in much the same way as physical assets. This value can be measured from the benefits returned from analytics services using the data. In organizations where data is not utilized productively, it can be considered a liability, and in particular a political liability when stakeholders know its potential is not being realized.
Customer data available from smart meters could be considered redundant to a network with widespread substation metering. However, customer data can add significant value to network planning investment by identifying changes in customer behaviors.
Contextual analytics drives quality investments
The predictive power of business data is contextual. Return on investment depends on evaluating the benefits of each improvement and with respect to the wider data strategy. Implementing initiatives, however, can be delivered as part of coordinated programs or small agile sprints and proof of concept projects.
The decision to undertake an analytics project should assess the relative strengths and weaknesses of the existing data, and consider whether the benefits of the initiative exceed the cost of remediation.
Understanding how data quality benefits analytics is key to right-sizing an improvement initiative and helps to justify the investment. As data quality improves, opportunities to implement powerful analysis and modeling techniques open up, leading to new insights and capabilities to deliver products and services.
Find out more about PSC’s partnership with Harmonic Analytics and contact us for more information about our Data Analytics services.
PSC is a proud member of Grid Forward. We share in their collaborative efforts to bring together all stakeholders to help modernize the electrical grid and accelerate innovation to reach our collective goals.